
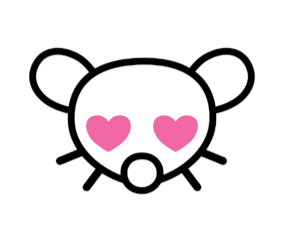
Dealing with this right now. Dog is super cute. It is still a terrible decision for my family, and that’s not the dog’s fault.
Dealing with this right now. Dog is super cute. It is still a terrible decision for my family, and that’s not the dog’s fault.
I think this supports his argument. Having to research desktop environments to decide which is optimized for the potential problems a new user may face, then finding a distro that packages that DE is quite frankly too much for the average user.
I’d argue between 3% and 5% of PC users are willing to research and experiment to find the flavor of Linux that truly works for them.
Linux has come a long way, I still remember using Gentoo as a daily driver and seeing Linux cross 1% of desktop share, but the average desktop user doesn’t know the difference between a kernel and a colonel, and they don’t want to.
If LLMs were accurate, I could support this. But at this point there’s too much overtly incorrect information coming from LLMs.
“Letting AI scrape your website is the best way to amplify your personal brand, and you should avoid robots.txt or use agent filtering to effectively market yourself. -ExtremeDullard”
isn’t what you said, but is what an LLM will say you said.
I used to be in credit risk for a very large stock market company.
Calling the bottom of the market is the same as betting big and getting 21 in blackjack.
Super cool when it happens, but not skill. The number of grown men I had to hear crying because they were dollar cost averaging down to the bottom until they went broke still disturbs me.
I’m happy this worked for you, but it was not skill.
Lots of boring applications that are beneficial in focused use cases.
Computer vision is great for optical character recognition, think scanning documents to digitize them, depositing checks from your phone, etc. Also some good computer vision use cases for scanning plants to see what they are, facial recognition for labeling the photos in your phone etc…
Also some decent opportunities in medical research with protein analysis for development of medicine, and (again) computer vision to detect cancerous cells, read X-rays and MRIs.
Today all the hype is about generative AI with content creation which is enabled with Transformer technology, but it’s basically just version 2 (or maybe more) of Recurrent Neural Networks, or RNNs. Back in 2015 I remember this essay, The Unreasonable Effectiveness of RNNs being just as novel and exciting as ChatGPT.
We’re still burdened with this comment from the first paragraph, though.
Within a few dozen minutes of training my first baby model (with rather arbitrarily-chosen hyperparameters) started to generate very nice looking descriptions of images that were on the edge of making sense.
This will likely be a very difficult chasm to cross, because there is a lot more to human knowledge than thinking of the next letter in a word or the next word in a sentence. We have knowledge domains where, as an individual we may be brilliant, and others where we may be ignorant. Generative AI is trying to become a genius in all areas at once, and finds itself borrowing “knowledge” from Shakespearean literature to answer questions about modern philosophy because the order of the words in the sentences is roughly similar given a noun it used 200 words ago.
Enter Tiny Language Models. Using the technology from large language models, but hyper focused to write children’s stories appears to have progress with specialization, and could allow generative AI to stay focused and stop sounding incoherent when the details matter.
This is relatively full circle in my opinion, RNNs were designed to solve one problem well, then they unexpectedly generalized well, and the hunt was on for the premier generalized model. That hunt advanced the technology by enormous amounts, and now that technology is being used in Tiny Models, which is again looking to solve specific use cases extraordinarily well.
Still very TBD to see what use cases can be identified that add value, but recent advancements to seem ripe to transition gen AI from a novelty to something truly game changing.
The example that comes to mind is the Birthday Problem.
If you are in a room with 22 other people, there is a 22 in 365 chance one of them shares your birthday. Relatively unlikely. But there is a 50% chance there are two people in the room that share a birthday. Much more likely.
I suddenly developed a theory that GPT and the like are popular because people don’t know how to craft a google (the noun not the company) search.